AI-powered security threat detection is revolutionizing cybersecurity by leveraging machine learning and automated security monitoring. These intelligent solutions enhance cyber threat intelligence, providing organizations with robust defenses against evolving internal and external threats.
While traditional security solutions play a crucial role, they often struggle to keep pace with the sophistication and sheer volume of modern cyberattacks. Here’s where Artificial Intelligence (AI) steps in, offering a powerful tool for combating cyber threats.
How AI Will Impact Software Security
AI-powered security threat detection is poised to revolutionize how software companies safeguard their systems, offering enhanced precision and efficiency in identifying and mitigating threats through AI-driven threat detection methodologies.
AI-powered Security
AI-powered security refers to the use of artificial intelligence (AI) and machine learning (ML) algorithms to automate and enhance various aspects of cybersecurity. It acts as a powerful defense mechanism for protecting systems, data, and networks from cyber threats. Here’s how AI is used in security:
- Threat Detection and Prevention: Machine learning in cybersecurity analyzes vast amounts of data (network traffic logs, user activity, system events) to identify anomalies and suspicious patterns that might indicate potential attacks. This includes:
- Advanced Malware Detection: AI can analyze file behavior and network traffic to identify novel malware strains that traditional signature-based detection might miss.
- Intrusion Detection: AI can monitor network traffic for suspicious activities like port scans, unauthorized access attempts, and denial-of-service attacks.
- User and Entity Behavior Analytics (UEBA): AI analyzes user activity logs to identify deviations from normal behavior patterns, potentially indicating compromised accounts or malicious insider activity.
- Automated Security Monitoring: AI automates repetitive tasks like log analysis, vulnerability scanning, and incident response, freeing up security professionals to focus on strategic initiatives and complex investigations.
- Cyber Threat Intelligence: AI can analyze threat intelligence feeds and combine it with internal data to provide a more comprehensive picture of the threat landscape, allowing for better threat prioritization and mitigation strategies.
AI-powered Attacks
While AI is primarily used for defensive purposes in cybersecurity, there’s growing concern about the potential for AI-powered attacks. Here’s how AI could be used offensively:
- Social Engineering: AI could be used to create more sophisticated phishing emails and social media scams, personalizing content to appear more believable and bypass traditional detection methods.
- Automated Attacks: AI-powered tools could automate large-scale cyberattacks, launching them faster and making them more difficult to defend against.
- Vulnerability Discovery: AI could be used to identify vulnerabilities in software and systems more efficiently, potentially giving attackers an edge over defenders.
It’s important to note that AI-powered attacks are still theoretical to a large extent. However, as AI technology continues to evolve, it’s crucial for cybersecurity professionals to be aware of these potential threats and develop strategies to mitigate them.
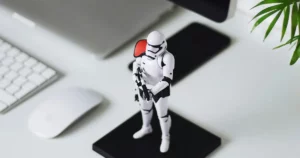
Compliance tools streamline risk assessments, manage regulatory changes, and integrate security testing. Photo by Liam Tucker.
Internal Threat Detection: AI as a Watchful Guardian
Internal threats can stem from employee negligence, compromised accounts, or even malicious insiders. We recomend three AI-powered solutions can be invaluable in identifying and mitigating these risks.
#1 User and Entity Behavior Analytics (UEBA)
AI analyzes user activity logs, identifying deviations from normal behavior patterns. This can reveal suspicious activities like unauthorized access attempts or data exfiltration.
Recommended for: Companies with a large user base or those concerned about insider threats.
How to Decide and Implement:
- Define Baselines. Analyze historical user activity logs to establish normal behavior patterns for different user groups (e.g., administrators, developers, customer support).
- Choose a UEBA Solution. Look for solutions that integrate seamlessly with your existing identity and access management (IAM) system and user activity logs. Evaluate features like anomaly detection, user risk scoring, and investigation tools. Top contenders are:
- Splunk User Behavior Analytics (UBA): Provides advanced analytics to detect anomalies and insider threats.
- Exabeam Advanced Analytics: Utilizes machine learning to identify risky behaviors and potential threats.
- LogRhythm UEBA: Integrates with SIEM to provide comprehensive threat detection and response.
- Securonix UEBA: Focuses on detecting advanced threats using behavior analysis.
- Microsoft Azure Sentinel: Offers integrated UEBA capabilities within its cloud-native SIEM solution.
- IBM QRadar Advisor with Watson: Uses AI and machine learning to enhance threat detection and investigation.
- Varonis DatAlert: Specializes in detecting insider threats and abnormal data access patterns.
- Darktrace: Uses machine learning to detect emerging threats across digital infrastructure.
- Continuous Refinement. Regularly review and update user baselines as user behavior and roles evolve. This ensures the AI model remains effective in identifying deviations from normal activity patterns.
How to be Most Successful:
- Educate Employees. Provide clear guidelines and training to employees on acceptable data access and usage practices. Foster a culture of cybersecurity awareness to encourage employees to report suspicious activity.
- Refine Baselines Continuously. As user behavior and roles evolve, update baselines to maintain the accuracy of anomaly detection.
#2 Data Loss Prevention (DLP)
AI-powered DLP solutions can scan data in motion and at rest, detecting sensitive information that shouldn’t be shared externally.
Recommended for: Companies handling sensitive data like financial information (check our dedicated article on fraud prevention), personally identifiable information (PII), or intellectual property.
How to Decide and Implement:
- Identify Sensitive Data. Classify the types of sensitive data you need to protect (e.g., credit card numbers, social security numbers, trade secrets).
- Define DLP Policies. Create DLP policies that dictate how sensitive data can be accessed, used, stored, and transferred (at rest, in motion, and in use).
- Choose a DLP Solution. Select a DLP solution that integrates with your data storage platforms (e.g., databases, cloud storage) and communication channels (e.g., email, file transfer). To name a few:
- Forcepoint Data Loss Prevention: Focuses on understanding user behavior to prevent data breaches.
- Digital Guardian Data Loss Prevention: Delivers visibility and control over data usage across the enterprise.
- Trend Micro Data Loss Prevention: Integrates with existing security infrastructure to protect sensitive information.
- Proofpoint Information Protection: Offers data discovery, classification, and protection capabilities.
- Check Point Data Loss Prevention: Prevents data breaches and data leakage across networks and endpoints.
- Cisco Data Loss Prevention: Integrates with Cisco’s security suite to provide comprehensive data protection.
- Varonis Data Security Platform: Monitors and protects data from internal and external threats with a focus on data access and usage.
How to be Most Successful:
- Regular Policy Review. Review and update DLP policies regularly to reflect changes in data types, regulations, and business processes.
- Educate and Train Employees. Educate employees on DLP policies and provide training on proper data handling procedures to minimize the risk of accidental data leaks.
#3 Anomaly Detection
AI algorithms can analyze vast amounts of data, including network traffic logs and system events, to identify unusual activity within the network. This might indicate malware infections or attempts to access unauthorized resources.
Recommended for:All companies, especially those with complex IT environments or a high volume of network traffic.
How to Decide and Implement:
- Define Baselines. Analyze historical network traffic logs and system events to establish baselines for normal activity. This includes metrics like login attempts, data transfers, and resource usage.
- Choose an Anomaly Detection Solution. Select an AI-powered anomaly detection solution that integrates with your network security monitoring tools and system logs. Look for features like real-time analysis, customizable alerts, and investigation tools. Options include:
- Exabeam Advanced Analytics: Utilizes machine learning to identify unusual user and device behaviors that may indicate threats.
- Securonix: Offers UEBA (User and Entity Behavior Analytics) capabilities with AI-driven anomaly detection for cybersecurity.
- LogRhythm: Uses AI and machine learning for real-time anomaly detection and threat hunting.
- Gurucul: Provides AI-based behavior analytics to detect insider threats and other anomalies.
- Rapid7 InsightIDR: Integrates AI and machine learning for detecting and investigating suspicious activities on networks and endpoints.
- Continuous Monitoring. Continuously monitor and refine anomaly detection models as your network, systems, and user base evolve. This ensures the AI stays effective in identifying new and emerging threats.
How to be Most Successful:
- Investigate All Anomalies. Promptly investigate all detected anomalies to determine if they represent a potential threat or simply legitimate unusual activity.
- Refine Detection Models. Based on investigation outcomes, refine your anomaly detection models to improve accuracy and reduce false positives.
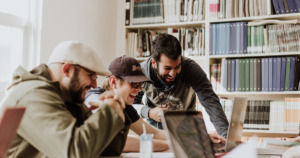
Your SecOps, Security Engineers, Security Analysts, and InfoSec professionals are the defenders of your digital fort, working tirelessly to protect your data, systems, and reputation.
External Threat Detection: AI as a Protective Shield
External threats encompass targeted attacks, malware deployment, and attempts to exploit system vulnerabilities. Cyber threat intelligence plays a crucial role in identifying these threats and bolstering defenses:
#1 Advanced Malware Detection
AI can analyze file behavior and network traffic to identify novel malware strains that traditional signature-based detection might miss.
Recommended for:All companies, especially those operating in high-risk industries or frequently targeted by malware attacks.
How to Decide and Implement:
- Choose an Anti-Malware Solution. Look for AI-powered solutions that utilize machine learning to identify novel malware strains based on behavior analysis. Ensure integration with your existing endpoint security software and network security tools for comprehensive threat coverage.You can choose from these and more:
- CylancePROTECT: Utilizes AI and machine learning to prevent malware infections by analyzing file behaviors and identifying malicious patterns.
- CrowdStrike Falcon: Offers AI-driven endpoint protection that includes anti-malware capabilities to defend against sophisticated attacks.
- Sophos Intercept X: Uses deep learning technology to provide advanced anti-malware protection for endpoints and servers.
- Palo Alto Networks Cortex XDR: Employs behavioral analytics and machine learning to detect and prevent malware across endpoints, networks, and cloud environments.
- Symantec Endpoint Protection: Uses AI-based threat prevention and analysis to defend against known and unknown malware threats.
- Maintain Updated Defenses. Regularly update malware definitions and threat intelligence feeds for your security software to ensure it can recognize the latest threats.
How to be Most Successful:
- Promote Security Awareness. Educate employees on cybersecurity best practices to help them identify and report suspicious emails or attachments. Phishing attacks are a common entry point for malware, so a vigilant workforce is crucial.
#2 Network Traffic Analysis
AI algorithms can continuously monitor network traffic, identifying suspicious patterns that might indicate ongoing attacks like botnet activity or phishing attempts. It can also automate vulnerability scanning and prioritize patching based on exploit likelihood and potential impact. This helps security teams focus on the most critical vulnerabilities first.
Recommended for: All companies, especially those with a large online presence or handling sensitive data transfers.
How to Decide and Implement:
- Choose an Network Security Solution. Select an AI-powered network traffic analysis solution that integrates with your network security monitoring tools and firewalls. Look for features like real-time analysis, anomaly detection, and deep packet inspection capabilities.
- Cisco Secure Network Analytics: Previously Cisco Stealthwatch, it uses AI and machine learning to provide visibility and threat detection across enterprise networks. It monitors network traffic to detect anomalies, threats, and potential breaches.
- ExtraHop Reveal(x): ExtraHop Reveal(x) uses AI-driven analytics to analyze real-time network data and detect threats such as malware, insider threats, and suspicious behaviors. It provides visibility into encrypted traffic and cloud environments.
- Gigamon ThreatINSIGHT: Gigamon ThreatINSIGHT integrates with AI and machine learning capabilities to analyze network traffic and detect threats across hybrid and multi-cloud environments. It provides real-time threat detection and response.
- FireEye Network Security: FireEye Network Security combines AI-driven analytics with threat intelligence to detect and block advanced threats. It uses machine learning to identify and respond to suspicious network activities and potential breaches.
- Configure Analysis. Configure the AI solution to analyze network traffic patterns for suspicious activities such as port scans, unauthorized access attempts, and data exfiltration attempts.
How to be Most Successful:
- Establish Baselines. Establish baselines for normal network traffic patterns based on historical data. This will provide a reference point for identifying significant deviations that might indicate an attack.
- Investigate Suspicious Activity. Promptly investigate all suspicious network activity to determine the source and potential impact. Take appropriate action to mitigate any identified threats.
#3 Vulnerability Scanning and Prioritization
AI can automate vulnerability scanning and prioritize patching based on exploit likelihood and potential impact. This helps security teams focus on the most critical vulnerabilities first.
Recommended for: All companies, especially those with a complex IT infrastructure or outdated systems.
How to Decide and Implement:
- Choose a Vulnerability Management Solution. Select an AI-powered vulnerability scanning solution that integrates with your asset management system to maintain an accurate inventory of all IT assets (hardware, software, applications).
- Qualys Vulnerability Management: Qualys provides a cloud-based vulnerability management platform that utilizes AI and machine learning to prioritize and remediate vulnerabilities across global IT environments.
- Tenable: Tenable offers Tenable.io, a vulnerability management platform that incorporates AI and machine learning to accurately prioritize vulnerabilities based on the risk they pose to the organization.
- Nessus Professional: Nessus, also developed by Tenable, is a widely-used vulnerability scanner that integrates AI capabilities to help identify, assess, and prioritize vulnerabilities across networks, systems, and applications.
- Nexpose by Rapid7: Nexpose is a vulnerability management solution that leverages AI and machine learning to provide visibility into vulnerabilities and prioritize remediation efforts based on risk.
- Skybox Security: Skybox Security offers a vulnerability management solution that uses AI and analytics to assess and prioritize vulnerabilities across hybrid IT environments, including virtual and cloud infrastructure.
- Configure Scanning. Configure the solution to scan your IT assets for known vulnerabilities based on industry databases and threat intelligence feeds.
How to be Most Successful:
- Maintain Asset Inventory. Regularly update your asset inventory to reflect changes in your IT environment (new devices, software deployments, etc.). This ensures all vulnerabilities are identified and addressed.
- Prioritize Patching. The AI solution should prioritize patching based on exploit likelihood and potential impact. Focus on patching critical vulnerabilities first to minimize the attack surface.
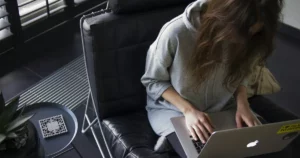
Strong APIs are the backbone of innovation, but weak ones are a hacker’s playground. Photo by Daria Nepriakhina 🇺🇦.
The Power of Combining Internal and External Threat Detection
The true strength of AI-powered threat detection lies in its ability to bridge the gap between internal and external threats. By analyzing data from both internal and external sources, AI can create a more holistic view of the security landscape. This allows you to identify potential insider threats collaborating with external attackers or external attacks attempting to exploit compromised internal accounts.
For example, AI might correlate unusual user activity (UEBA) with suspicious network traffic patterns (network traffic analysis) to identify a potential insider attempting to exfiltrate data. This comprehensive approach significantly enhances your ability to detect and respond to sophisticated cyberattacks.
Implementing AI-Powered Threat Detection
Several reputable AI-powered threat detection solutions are available. Here are some key considerations when making your choice:
- Scalability: Ensure the solution can handle the volume of data your company generates.
- Integration: Choose a solution that integrates seamlessly with your existing security infrastructure.
- Alert Fatigue Management: AI can help reduce false positives, but a well-designed solution should also prioritize critical alerts to avoid overwhelming security teams.
- Compliance Alignment: Ensure the solution adheres to relevant industry regulations for data privacy and security.
AI-Powered Security Threat Detection: Your FAQs Answered
This FAQ explores common questions about AI-powered threat detection and its role in enhancing cybersecurity for software companies:
A Proactive Approach to Cybersecurity
By leveraging AI-powered threat detection, software companies can move from a reactive to a proactive security posture. This allows you to identify and address threats before they can cause significant damage using automated security monitoring. As cyber threats continue to evolve, AI will play an increasingly critical role in safeguarding your organization’s data and maintaining a secure environment.
Remember, AI is a powerful tool, but it’s not a silver bullet. A comprehensive cybersecurity strategy should combine other AI resources to machine learning in cybersecurity with other security measures like employee training, strong access controls, and regular security assessments. By deploying a layered security approach and adopting AI-powered threat detection, software companies can build a robust defense system and achieve their business goals with confidence.
At Ubiminds, we specialize in finding the right professionals for SecOps teams and have the expertise to help companies implement AI capabilities within their SDLC. Reach out to us to learn more about how we can support your cybersecurity initiatives.
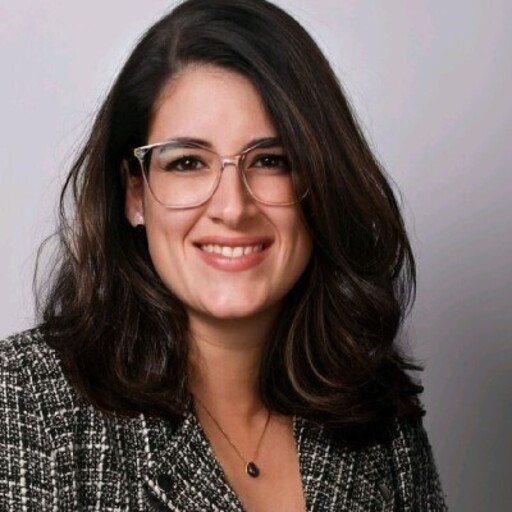
International Marketing Leader, specialized in tech. Proud to have built marketing and business generation structures for some of the fastest-growing SaaS companies on both sides of the Atlantic (UK, DACH, Iberia, LatAm, and NorthAm). Big fan of motherhood, world music, marketing, and backpacking. A little bit nerdy too!